Automation, Augmentation, Value Creation & the Distribution of Income & Wealth
Digital technologies are transforming the economy and society. The dimensionality and scope of the impacts are bewildering and too numerous to cover in a single essay. But of all the concerns around digital technology (and there are many), perhaps none has attracted more attention, and generated deeper anxiety, than the impact of various types of automation on work and on the structure of the economy. I focus on the ways in which the digitization of virtually all data, information, and content is transforming economies. And more specifically, I look at the impacts of automation, augmentation, AI, machine learning, and advanced robotics on economic transformations, on work, and on the distribution of income and wealth.
Digital technology can be thought of as digital machines (computers, servers, and various other portable devices), software, and networks (with standardized protocols) creating, storing, operating on, and transmitting information in digital form. “Use cases” refer to applications of digital technology such as mobile payments, social media, online commerce, and location-specific services like maps.
Automation and digital machine augmentation are a class of use cases. Automation involves replacing people with machines in the performance of certain tasks that machines can carry out without human intervention or guidance. Augmentation connotes adding machines to a work environment, enabling people to be more productive.1 The two As are flip sides of the same coin.
Augmentation of human productivity using machines is hardly new. If you think of tools as simple machines, augmentation of human capabilities has characterized most of human history on the planet. In the first industrial revolution, when energy and power were added to the mix via steam engines, and later electricity and fossil fuels, machine augmentation produced (with a lag) a huge, sustained acceleration in productivity. We called it mechanization. And it changed work, raising concerns that there would not be enough jobs to go around, or that a subset of people would not be able to learn how to do the new jobs that required working with machines.
This kind of machine augmentation can produce transitory unemployment for a few reasons. First, work in the sectors experiencing rapid machine augmentation requires new sets of skills, and these do not adjust immediately in the workforce. Second, utilizing the new technology often requires the installation of new production systems and business models, which again is far from instantaneous. And third, increased productivity will give rise to rising incomes, but that may not result in higher demand right away. For instance, at present, labor incomes in many countries are diverging from productivity for much of the population; income is going to capital and toward the wealthy end of the income spectrum, where savings are higher.
That said, longer term, two parallel processes have typically prevented permanent unemployment from becoming a reality. Incomes rise, and with that increase comes elevated demand for goods and services. Second, with higher incomes, labor markets adapt to different work-leisure trade-offs; hours worked steadily decline over long periods of time. Data on hours worked support this proposition. Across countries, hours worked per year declines with per capita income, and across time among Organisation of Economic Co-operation and Development countries, hours worked declines over time.2 (See Figure 1.) In the pre-industrial era, many people worked long hours just to provide for basic needs.
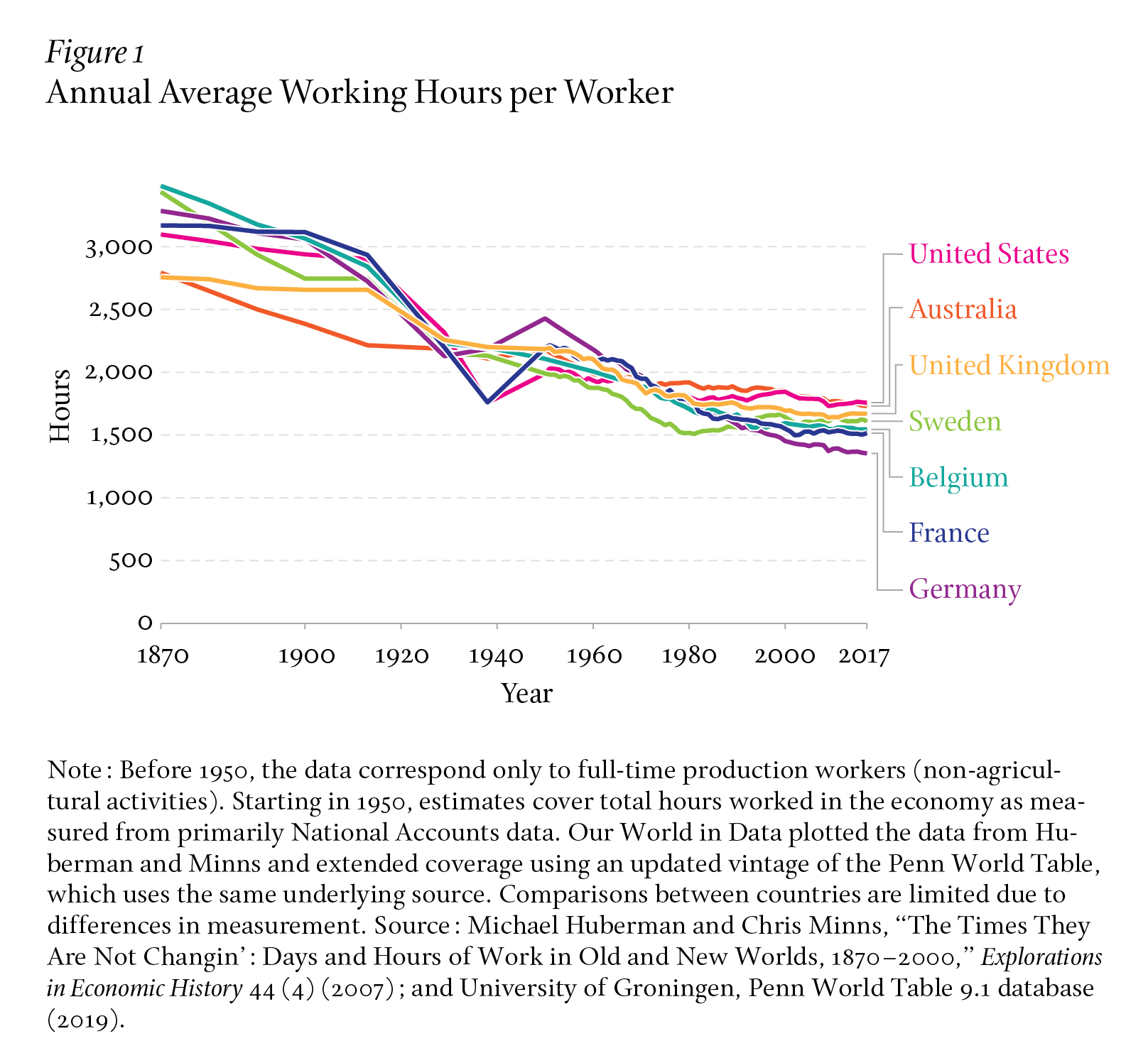
Nevertheless, concerns about employment in the aggregate–in effect, whether there are enough jobs to go around–have been common in periods of rapid technological advancement.
In the predigital era, the issue was not about what might be called full automation, because machines did not perform tasks all by themselves. Mechanization was the focus of attention. In modern parlance, one can think of it as machine augmentation, but not digital machines. People used machines to do tasks much more quickly, often with higher-quality outputs, and even to produce things that were impossible in the premachine age.
Admittedly, the historical line between mechanization and automation is sometimes a little blurry. The history of weaving machines or looms contains fascinating examples of predigital automation.3 But for the most part, in the predigital era, machines did not carry out long and relatively complex sequences of actions without human intervention. In general, machines augmented and replaced humans in the physical performance of tasks, but the information and control layer, governing the sequencing and timing of the activities of machines, remained firmly in human hands or minds. Machines in the industrial age were powerful but they did not function autonomously. In the digital era, this is no longer true.
This somewhat simple fact helps explain why we find ourselves in uncharted territory. In any economy there is a very large collection of activities that involve gathering, recording, analyzing, and transferring information, transactions, coordination of activity, pattern recognition, and decisions. There is an enormous amount of embedded knowledge in an economy, and sophisticated transmission mechanisms via institutions and informal networks that support information flows and decisions (large and small) that in the aggregate determine the performance of the economy.
Which brings us to automation, digital machines, and software. Early computers were programmed manually to perform a specific function, with wires, somewhat like an old telephone switchboard.4 That cumbersome and limiting technology was quickly replaced by programmable computers, which load a set of digital coded instructions and then execute them autonomously. The code contains the logical sequence of steps that would be carried out by humans were they performing the task. Up until recent advances in artificial intelligence (over the past decade), this has been the basic model of digital automation.
Before the programmable computer and the digitization of most information in the form of data, automation and augmentation in the information, control, coordination, decision, and transactions layers of the economy were negligible. Now, as of about the past sixty years, for the first time we have powerful machines in the information, coordination, and decision (ICD) layer. That is in part why digital economists Erik Brynjolfsson and Andrew McAfee referred to this as the second machine age in their influential book of the same name.5
For those who might have suspected that this ICD layer was perhaps an important but relatively minor (in terms of value-added) part of the economy, the effect of the COVID-19 pandemic on the economy was revealing. Substantially disconnecting the functioning of the ICD layer from the need for physical proximity, which is what in part modern network-based digital technology has done, enabled economies to keep operating with substantially reduced mobility and physical contact. Of course, there are important sectors in which proximity is unavoidable that either contracted or shut down during the pandemic or stayed open but at considerable health risk to their workers.
In short, the ICD layer, which is effectively the governing and control mechanism in the economy and the market system, now has powerful machines that automate, replace, and sometimes outperform people in some tasks, while simultaneously augmenting people in the performance of other tasks.
It is tempting to assume that the second machine age will follow a pattern like industrialization, and indeed it may. But as we are in somewhat uncharted territory, we should not rush to this conclusion. While the impact of digital technology has already been substantial, the technology seems clearly set to continue to advance.
The full economic impact of AI and machine learning, for example, is largely in the future. The pandemic economy has accelerated digital adoption across a range of sectors, including many that had been lagging in this respect.6 Powerful machine learning tools are now widely available for a rapid digital transformation.
The first round of digital automation involved codifying tasks. Codifiable tasks have two properties: 1) people can do them–meaning, carry out the steps and 2) we can figure out and precisely describe the logical steps we use in performing them, steps and logic that are then embodied in code. It is the second part that enables digital machines to automate significant parts of the information-processing and control layer of the economy. It is also the part that blocked progress in artificial intelligence until the machine learning revolution, because a variety of “tasks”–such as image recognition, understanding natural language, translation, and in fact a number of pattern-recognition and prediction capabilities that humans have–defied codification. This impasse in AI put quite severe limits on the scope for automation.
AI based on machine learning broke this impasse essentially with an end run. Take image recognition, an area that has experienced dramatic advances in the past ten years. Instead of trying to find a set of “rules” for classifying images, deep learning algorithms analyze millions of digital images to detect patterns. Over time, the predictions about images become increasingly accurate. Similar advances are occurring in speech, language translation, a host of medical applications, and many more fields.
For the economy, the significance of the breakthroughs in AI via machine learning lies in dramatically increasing the scope for automation and, critically, augmentation across the entire economy. Advanced robotics, autonomous vehicles, radiology, analysis of DNA sequences in relation to diseases, reading vast amounts of literature for doctors and other professionals, expanding access to credit and other financial services via algorithms that close informational gaps are all enabled by machine learning. And these are just a few examples.
The first round of automation, now in its middle age, was not AI-driven but required codification of tasks. We have data on its impacts. This second round of automation and augmentation enabled by machine learning is in its early stages. Its full impact is not yet apparent in economic data.
The impact of the codification version of automation has already been substantial. Routine white- and blue-collar jobs, or more precisely jobs in which routine tasks are a large component, began declining with a noticeable acceleration around the year 2000. Here, routine refers to jobs that substantially consist of tasks that are codifiable and hence subject to partial or complete automation. Codifiable, however, does not mean simple. The sequence of steps including conditional branching, true/false determinations, and classification can be long and complex.
Since many of these routine jobs were associated with mid-level incomes, the first order effect in economic terms has been to reduce employment in routine, middle-income jobs. A distinctive characteristic of digital automation is that it includes both blue- and white-collar work, the latter involving processing, storing, recording, and retrieving information.
A resulting pattern of job and income polarization has been documented for most of the developed economies.7 The immediate effect on the income distribution is to flatten it, making the tails larger and the middle smaller. Of course, if the displacement of middle-income jobs were extreme, we could start to see bimodal distributions, but that has not yet happened.
How does the economy respond when a certain class of jobs is automated? Initially, there may be transitory unemployment, and adjustment typically takes place dynamically as follows. At first, people will look for jobs that match their current specific skill set. But that does not work for everyone because those jobs are declining in the economy. They then set employment as a priority and move to jobs in which the skill requirements are either fewer, or are more easily acquired quickly, as we have seen with part-time jobs in the Internet-enabled gig economy. Often that means lower incomes. What one sees from a macroeconomic perspective is not necessarily large-scale increases in unemployment, but rather a deterioration in the income distribution. One can think of this as a shifting equilibrium, assuming the skills on the supply side remain fixed.8
However, that is not the end of the story, because the skills and human capital side of the job market are not fixed, just slower moving. People look for and start investing in skills and human capital that are in demand in nonroutine job categories with higher incomes. The pace of this more time-consuming process crucially depends on the presence or absence of supporting institutions, including employers. That is why, for example, there are numerous partnership initiatives in the United States, involving government at all levels, businesses, and educational institutions to accelerate the skills-transition process. And in many other countries, the existing and well-developed educational and skills-training infrastructure is being adapted to digital transformations to continue to play a key role in these supply-side skills transitions.
A second key gatekeeping factor with respect to the skills transition is the distribution of income and wealth. Investing in one’s own human capital takes time and financial resources regardless of the quality of the institutional support mechanisms. If income and wealth inequality is extreme, then the lower part of the income/wealth distribution will struggle to make the investments in their own human capital, the more so in the absence of high-quality publicly funded and low-cost key public services.
Notice the circularity here. Automation of middle-income jobs has contributed to suppressing the middle of the income distribution. For those who are pushed toward lower deciles, the challenge of investing their way out via new skills acquisition becomes more difficult. So the income distribution is both an outcome of and an input to the digital transitions in work. The appropriate conclusion seems to be that policies that directly address high income inequality will turn out to contribute to successful work transitions, even if that is not the primary purpose of the policies.9
The skills-adjustment process can have a beneficial effect on the income distribution. Essentially, it partially undoes the adverse initial distributional effect of automation by increasing the supply of people with skills that are in high demand and have higher incomes. In fact, other things equal, it may lower the incomes in these higher-skill/income segments, or the so-called skills premium. But it also reduces the supply of people in the lower-skill/income part of the spectrum, and hence puts upward pressure on the wages there.
A natural and important question is, “Will the skills-adjustment process largely eliminate the adverse initial distributional effects of automation?” An honest answer, I think, is that we do not know because we have no way of determining with any precision, ultimately, how fungible the human capital stock really is in the population. The existing evidence does not support the view that adverse distributional effects are transitory. To date, skill-biased technical change (substantially in the digital area) looks like it has shifted income upward in the distribution, even after the skills-adjustment process on the supply side is well underway.10
The main takeaways here are: 1) we are grappling with complex structural changes and transitions in work, skill requirements, and human capital, not equilibria; 2) the purpose and end point of this transition is to turn automation into digital augmentation; 3) technology is not stationary with the result that the target keeps moving, especially with the application of machine learning across most sectors of the economy; and 4) extreme income inequality combined with institutional and policy shortfalls risk turning a complex transition into a trap for the lower-income part of the population.
Two other dimensions of the challenge of AI and automation deserve attention. One is that technology can and does adapt in ways that reduce the magnitude of the skills-acquisition problem. We see this all around us. Digital equipment and systems are designed to be easier to use, and markets reward that kind of innovation. Perhaps the best historical example is the graphical user interface (now found on virtually every consumer digital device). It is so pervasive that we all take it for granted and for younger people, it is simply the normal way to interact with digital machines. But there was a time when interacting with “computers” was a lot less intuitive, and largely confined to those with the requisite training.
Let me turn now to AI and machine learning technologies. As noted earlier, machine learning implies a vast expansion in the tasks or subtasks that can be automated: for example, advanced robotics, autonomous vehicles, and reading and editing technical literature for, say, medical professionals. In addition, machine learning–based pattern-recognition applications go well beyond human capabilities in some areas, as applied to genetics and biomedical science, for example, taking it well beyond automation and firmly into the realm of augmentation.11
Since these advances in AI for work entail a significant expansion of tasks or subtasks that can be automated and performed by machines, one can ask where on the income spectrum these work-related disruptions will land. Again, an honest answer is that it is too soon to know with any confidence. But a reasonable guess is across the board, and not mainly in the middle-income range of white- and blue-collar work, as in the first round of code-based digital automation. At one end, low-income labor-intensive manufacturing in developing countries is set to be disrupted in a way that was not possible before the recent advances in AI and robotics, and that presents challenges to the development model of low-income countries. On the other end, scientific research and technology development and high-end professional services look to be within the target range as well, not in the sense of full automation, but rather in substantial digital machine augmentation, via the automation of key tasks.
Thus far, we have focused mainly on various aspects of the digital transformation in developed economies. But their impact does not stop there. There are at least two important classes of developing economies in which the impact of automation and augmentation has large and diverse current and future impacts: middle-income countries (often called emerging economies) and the lower-income countries in which the growth and development process is in the early stages.
Emerging economies are developing countries that have reached middle-income levels. Some are growing quickly while others struggle with growth. But for the most part, they have resources and, in general, reasonably well-developed digital infrastructure. But they still have poor segments of the population in need of informational and related services. Many live outside major urban areas with limited access to traditional offline services. In these economies, probably more than either developed or lower-income economies, the digital transformations are a large net positive in multiple dimensions. Generally, these economies have left behind the labor-intensive manufacturing and assembly growth and employment engines that are threatened by modern AI and advanced robotics and that represent a significant obstacle for lower-income countries seeking to replicate the high-growth patterns achieved by their predecessors.
In the emerging economies, e-commerce, mobile payments, and fintech–all now powered by AI–are closing the service availability gaps associated with remoteness. The same is true in education and health care. In addition, the lower-income parts of the population have traditionally had difficulty accessing modern services because of the absence of documentable identities and financial track records. I call this the anonymity problem. Digital data and machine learning are proving to be powerful tools for bridging these gaps and overcoming the obstacles.
And on the employment front, the middle-income economies are in complex transitions in which the service sectors are expanding as a share of the economy and employment. Generally, the digital transformations are accelerating these changes and creating considerably more jobs than are being eliminated. This is not to say the previous discussion of digital transformations and work are entirely different here. There are skills transitions to be navigated, too. But the balance is different. It is more about training than retraining. Job losses associated with automation are small in relation to the job losses in the declining labor-intensive sectors. Indeed, automation is seen as a way to keep manufacturing sectors that are transitioning from labor-intensive to digitally capital-intensive. These are sectors that, in the absence of the productivity increases that go with automation, would migrate to lower-income parts of the global economy. In addition, legacy systems that tend to hold back the pace of change in developed economies are less developed in emerging economies. So the new digitally based economy, as it emerges from the advances in technology and use cases, is in many ways embraced faster. Technologically, they are leapfrogging intermediate steps.
There is also a very interesting trend in global entrepreneurial activity that is directly relevant to employment and structural change in emerging economies: a rapid increase in new company formation across a range of emerging economies and continents. It is linked in large part to the rapid spread of the digital economy. The financial and other parts of innovative ecosystems that support this have also become global in coverage. The result is high-growth companies and unicorns (privately held startup companies with a value of over $1 billion) proliferating across the globe. (See Table 1.)
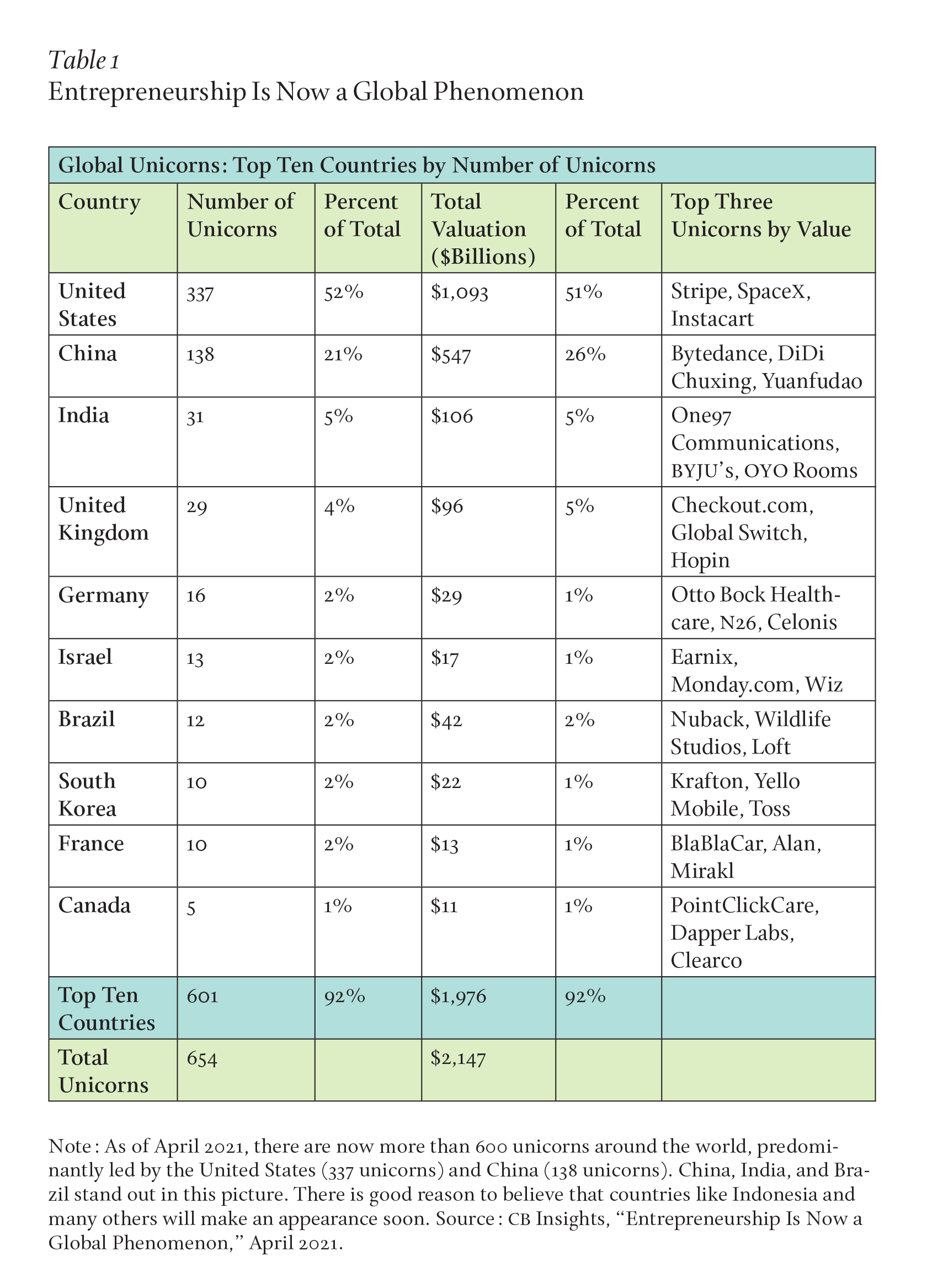
As this trend gains momentum, it provides powerful new employment engines and entrepreneurial opportunities, especially for younger parts of the population. The opportunity is created in part by automation in the development and improved performance of new markets and complementary systems. These systems are increasingly architected, modified, and improved by AI. This dimension of automation is much less discussed than automation in the context of work, but it is an important element of AI-powered automation and augmentation. At least in the digital area, the entry barriers are low and the initial capital requirements are also low, making them accessible, ideal environments for fostering innovation, entrepreneurship, and new company formation.
For the lower-income countries, the picture is similar in some respects but different in others, presenting a challenge and an opportunity. The mobile Internet has substantially closed the gap in terms of basic digital infrastructure, though there is more investment needed to bring coverage, network capacity, speed, and reliability up to middle-income-country levels. For these economies, a growth model is needed that leverages global economy demand and technology. Traditionally, for non-resource-rich countries, the core of the growth model has been exports of labor-intensive goods and process-oriented manufacturing and assembly. It is a model based on low labor costs and has historically had the virtue of being a powerful employment engine.
The problem is that AI and advanced robotics are making inroads into the growth and employment model that depends on low-cost labor, in which the comparative advantage lay in labor-intensive manufacturing (think textiles and apparel). This aspect of automation is making labor costs much less influential in determining where to locate production. It is leading to what economist Dani Rodrik has termed premature deindustrialization. The problem is that it is thus far not clear whether there is an equally capable alternative engine to power growth and development.
That said, the benefits in terms of digitally enabled inclusive growth patterns, described above for emerging economies, including especially expanded opportunity for entrepreneurial activity, also apply to the lower-income countries, provided the digital infrastructure is in place. The importance of this should not be understated for fostering inclusive growth patterns.
How you think about these issues depends on the unit of analysis. If the unit is a working person, then automation can eliminate the job. More commonly, though, the result is augmentation: it replaces part of the job, changing the nature of the work. On the other hand, if you start with the unit being some subsystem of the economy, say a manufacturing facility, then machines are essentially augmentation, just as they were in the industrial revolution, and automation, which is new and digitally enabled, is an important and powerful tool in making us more productive.
© 2022 by Michael Spence. Published under a CC BY-NC 4.0 license.